Imagine you’re a cybersecurity professional dealing with an unprecedented volume of threats. With AI and ML, you’re not just reacting to attacks; you’re proactive. These technologies empower you to detect threats in real-time, analyze vast amounts of data for patterns and anomalies, and respond instantly. You can automate routine security tasks, freeing you to focus on strategic threat analysis and incident response. But as you integrate AI into your security operations, new challenges emerge. How do you guarantee AI-driven systems are secure and trustworthy? Let’s explore these transformations and the implications they hold for your cybersecurity efforts.
Advancements in Threat Detection
Advancements in Threat Detection:
You’re facing a challenging task: keeping up with the ever-evolving landscape of cyber threats. Artificial intelligence (AI) and machine learning (ML) are transforming threat detection by giving you the edge you need to stay ahead. These technologies can analyze vast amounts of data in real-time, identifying patterns that human analysts might miss. By leveraging AI and ML, you can detect threats more quickly and accurately, reducing the risk of successful cyberattacks. This is vital for strong security standards and governance frameworks that protect sensitive data.
AI-powered tools can automatically scan your networks and systems for vulnerabilities, predict potential attacks, and even respond to incidents autonomously. For instance, AI algorithms can analyze network traffic, user behavior, and system logs to identify suspicious activities, such as ransomware attacks. Furthermore, AI-driven threat detection can help you prioritize risks, focusing on the most critical vulnerabilities and potential threats. By embracing AI and ML, you can enhance your cybersecurity posture, protect sensitive data, and prevent costly breaches. This proactive approach is critical, as the stakes are high, and the consequences of inaction can be disastrous.
Automating Cybersecurity Processes
Building on the enhanced threat detection capabilities provided by AI and ML, you can take the next step by automating your cybersecurity processes. This not only streamlines your security operations but also greatly bolsters your defenses against increasingly sophisticated cyber attacks.
With AI-driven automation, you can rapidly respond to threats by leveraging algorithms that analyze vast volumes of data to identify potential vulnerabilities and anomalies. For instance, AI can automatically block malicious IP addresses and even automate incident response workflows to mitigate breaches more effectively. Additionally, AI-powered systems can continuously scan your infrastructure to uncover weaknesses that could be exploited by attackers and reduce the risk of successful cyberattacks.
AI’s Role in Zero-Day Exploits
In the ever-evolving landscape of cybersecurity, AI and ML technologies are essential in addressing one of the most challenging vulnerabilities. These are attacks that exploit software vulnerabilities that have not been previously identified making them particularly dangerous. Traditional security measures often struggle to detect zero-day threats because they lack the necessary intelligence to recognize novel attack vectors.
AI and ML come into play by analyzing vast amounts of data to identify a zero-day exploit. These technologies can process and analyze data in real-time and response to threats that conventional systems might miss. For instance, AI-powered systems can scan code and configurations to uncover vulnerabilities that could be exploited by attackers. This proactive approach not only helps in preventing attacks but also aids in patching vulnerabilities before they become a risk. By integrating AI and ML, cybersecurity teams can enhance their defenses against these unpredictable threats, providing a critical layer of security in the ongoing battle against cyber threats.
Machine Learning in Cyber Defense
You’re facing an urgent challenge in cybersecurity, one where machine learning (ML) emerges as a critical tool in enhancing threat detection and automating risk assessment. By leveraging ML algorithms that learn from historical data, you can considerably improve your ability to detect anomalies, analyze malware, and evaluate user behavior analytics in real-time, thereby bolstering your cyber defense strategies. Additionally, integrating ML into your cybersecurity system allows for a more proactive approach, enabling you to identify and respond to potential threats before they escalate into major incidents.
Enhanced Threat Detection
Machine learning in cyber defense has revolutionized threat detection by leveraging advanced algorithms to identify and predict potential threats. You’re now capable of analyzing vast amounts of data at unprecedented speed, detecting anomalies that were previously undetectable by traditional security tools. This results in enhanced threat detection, giving you a proactive edge against cyber adversaries.
With machine learning, you can identify patterns and anomalies indicative of malicious activities, such as data exfiltration attempts or zero-day exploits. AI algorithms can continuously monitor your network and system logs, automatically identifying and flagging suspicious behaviors that may indicate a threat. Furthermore, machine learning enables you to anticipate potential threats by studying historical data and attack patterns, allowing you to implement preventive measures before an attack occurs.
This predictive capability is essential, as it allows you to stay ahead of evolving cyber threats. By integrating machine learning into your cyber defense strategy, you can greatly bolster your threat detection capabilities, reducing the risk of successful cyberattacks and protecting your critical data assets.
Automated Risk Assessment
Through the integration of machine learning in cyber defense, automated risk assessment becomes a powerful tool in your arsenal against cyber threats. By leveraging AI algorithms, you can continuously scan your network, systems, and applications to identify potential vulnerabilities, thereby greatly reducing the risk of successful cyberattacks. Machine learning models are trained on historical data, allowing them to learn from past threats and adapt to new ones, ensuring robust and proactive protection.
Automated risk assessment not only detects vulnerabilities but also predicts potential threats by analyzing patterns and anomalies in real-time data. This enables cybersecurity teams to prioritize mitigation efforts efficiently, allocating resources to the most critical vulnerabilities first. For instance, AI can identify risky user behavior, flag suspicious login attempts, and alert on potential malware infections, all in real-time. By automating this process, you can respond to threats faster, minimizing the time window for attackers to exploit vulnerabilities. This proactive approach empowers you to stay ahead of adversaries, safeguarding your valuable assets and enhancing your overall cybersecurity posture.
Enhancing Incident Response Times
Incident response times are essential in minimizing the damage caused by cyberattacks. You need to act quickly to prevent further harm, and that’s where AI and ML come in. By leveraging these technologies, you can greatly enhance incident response times. AI algorithms can analyze vast amounts of data in real-time, identifying potential threats and triggering immediate responses. This means you can detect and address cyberattacks much faster than traditional methods allow.
For example, AI-powered incident response platforms can automatically investigate workflows, identify the root cause of an incident, and suggest appropriate remedial actions. This automation empowers your security team to respond quickly, minimizing the impact of security breaches. Furthermore, AI-driven solutions can learn from historical data, improving their effectiveness over time. They can analyze network traffic, user behavior, and system logs to identify suspicious activities, enabling you to respond promptly and effectively. With AI and ML, you can optimize your incident response strategy, protecting your valuable assets from ever-evolving cyber threats. This integration is vital for staying ahead in the cybersecurity landscape.
The Future of Cybersecurity Tools
The Future of Cybersecurity Tools
As the cybersecurity landscape continues to evolve, the tools designed to protect it must also step up. You’re facing unprecedented threats, and traditional methods alone can’t keep pace. This is where AI and ML come into play, revolutionizing the way you defend against cyberattacks.
You need to leverage AI’s ability to process vast amounts of data and identify complex patterns. This enables the detection of sophisticated threats in real-time, greatly reducing the window for attackers to exploit vulnerabilities. Machine learning algorithms can also predict attacks, allowing you to proactively strengthen defenses.
Moreover, AI-driven cybersecurity tools can automate incident response, minimizing the time spent on detection and response. This is essential, as every second counts in the event of a cybersecurity breach. By integrating AI and ML into your cybersecurity arsenal, you can stay ahead of emerging threats and protect your valuable assets effectively.
Incorporating AI and ML isn’t just about adopting new tools; it’s about transforming your cybersecurity strategy to meet the challenges of the future. This proactive approach is vital for safeguarding your data and systems in an ever-evolving threat landscape.
Cybersecurity Challenges and Risks
You face significant cybersecurity challenges and risks, particularly in vulnerability detection, threat prediction, and human error management. The integration of custom software solutions can enhance your organization’s ability to detect vulnerabilities in real-time, but AI and ML solutions can be compromised if not properly trained, leading to false positives or undetected threats. In addition, these technologies also pose risks if not managed correctly, which can exacerbate the very cybersecurity threats they aim to mitigate.
Vulnerability Detection Challenges
Detecting vulnerabilities in cybersecurity is a challenging task that has become increasingly complex with the rapid evolution of threats. You face a landscape where attackers are leveraging AI and ML to exploit vulnerabilities faster and more effectively. This dynamic environment necessitates a proactive approach, leveraging AI and ML for vulnerability detection to stay ahead of threats.
Using AI and ML, you can automate the process of identifying vulnerabilities by scanning code, examining applications, and analyzing network data. These technologies can spot weak spots in codes or incorrect configurations, helping you implement necessary repairs. AI can also analyze attack data to predict potential future assaults, allowing you to apply restraint measures against possible threats in time. However, challenges remain, particularly in ensuring that AI systems are trained on accurate and thorough data to avoid false positives and negatives. Additionally, the constant need to update and fine-tune these systems to keep pace with evolving threats is a significant challenge. Effective vulnerability detection requires continuous monitoring and adaptation to maintain a robust cybersecurity posture.
Threat Prediction Risks
Leveraging AI and ML for vulnerability detection sets the stage for addressing another critical challenge in cybersecurity: threat prediction risks. You’re not just identifying vulnerabilities; you’re anticipating the tactics and strategies attackers might employ. AI and ML can analyze vast amounts of threat intelligence data to predict potential threats, but this comes with significant risks.
These technologies, while powerful, are not infallible. Predictive models can be misled by biased data or sophisticated adversarial tactics. Hackers may exploit these vulnerabilities to avoid detection or create false positives, overwhelming your security systems. Additionally, AI-driven threat prediction relies on high-quality, diverse data; without it, your predictive capabilities are compromised.
Furthermore, AI and ML can be turned against you. Attackers might use these technologies to devise mutating malware or evade detection. This risk underscores the need for robust, AI-driven threat prediction strategies, coupled with continuous human oversight and data integrity measures. By addressing these challenges, you can harness the power of AI and ML to predict and prevent threats, but it requires a vigilant and proactive approach to cybersecurity. Effective use of these technologies demands a deep understanding of both their capabilities and limitations.
Human Error Management
Human Error Management in Cybersecurity: The AI and ML Impact
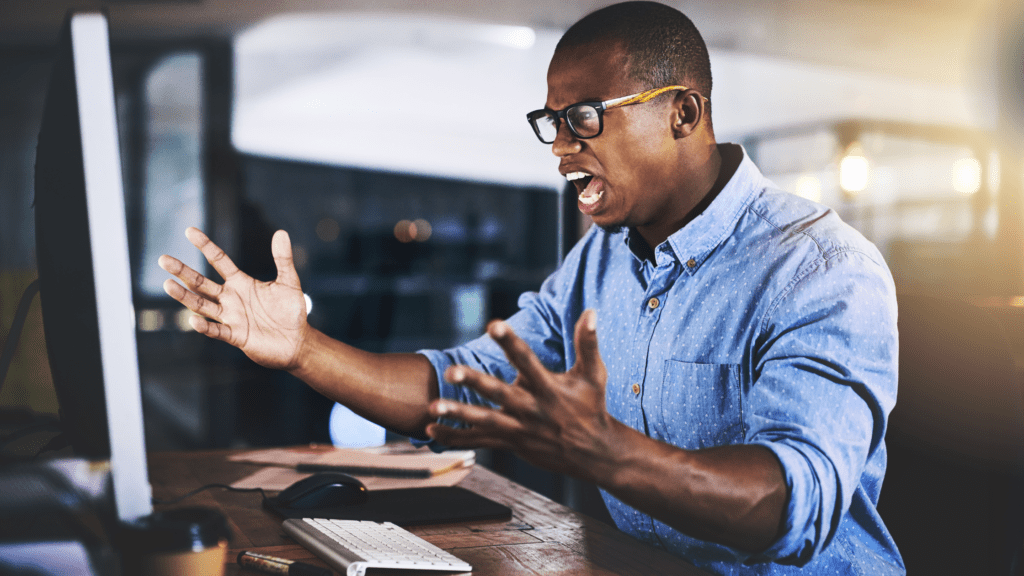
In the complex landscape of cybersecurity, human error management stands out as a considerable challenge. You face a formidable task in mitigating risks arising from manual configuration, repeated activities, and the inevitable missteps of human operators. The introduction of Artificial Intelligence (AI) and Machine Learning (ML) into cybersecurity is transforming this landscape by greatly reducing the impact of human error.
Traditional cybersecurity methods heavily rely on manual processes, which are not only time-consuming but also prone to errors. For instance, configuring systems, updating software, and responding to threats manually can lead to misconfigurations, overlooked vulnerabilities, and delayed responses, all of which hackers can exploit. AI and ML technologies automate these tasks, minimizing human intervention and the associated risks. These technologies analyze patterns and learn from past data to predict and prevent future threats, reducing the likelihood of mistakes that manual processes might introduce.
Ethical Considerations of AI
As you explore the domain of artificial intelligence, it becomes essential to contemplate the ethical implications that accompany its use. The integration of AI and ML in cybersecurity, while offering unprecedented benefits, also raises critical ethical considerations. You must be aware that AI systems can be manipulated by malicious actors, leading to unintended consequences. For instance, adversarial attacks can deceive AI models, causing them to produce incorrect outputs or make incorrect decisions, which can compromise cybersecurity defenses.
Moreover, the reliance of AI models on large datasets for training poses significant ethical concerns. If these datasets are contaminated with biased or manipulated data, it can impact the performance and behavior of the AI system, potentially leading to biased or inaccurate results. This underscores the importance of guaranteeing proper data governance and implementing privacy-preserving AI techniques to maintain a balance between security and privacy. It is imperative that you address these ethical considerations proactively to safeguard against potential vulnerabilities and guarantee the responsible use of AI in cybersecurity.
Improving Cybersecurity Efficiency
Enhance your cybersecurity posture by integrating AI and ML into your defense strategies. By leveraging these technologies, you can markedly improve the efficiency of your cybersecurity efforts. AI-driven systems can analyze vast amounts of data to detect and anticipate threats, enabling rapid response times and minimizing the impact of potential breaches.
With AI and ML, you can automate routine manual tasks, reducing human error and increasing the effectiveness of your cybersecurity team. Machine learning algorithms can scan infrastructure, code, and configurations to identify vulnerabilities, allowing you to proactively address potential weaknesses and reduce the likelihood of successful cyberattacks.
Moreover, AI-powered incident response platforms can automate investigation workflows, rapidly identify the root cause of incidents, and suggest appropriate remedial actions. This capability empowers your security team to respond quickly and precisely, minimizing downtime and protecting sensitive data. By embracing AI and ML in your cybersecurity strategy, you can enhance your defenses and safeguard your valuable assets more effectively.
AI and Cybersecurity Integration
You’re entering a critical phase in cybersecurity where the integration of artificial intelligence (AI) and machine learning (ML) technologies becomes indispensable. By leveraging AI and ML, you can markedly boost the efficiency of your cybersecurity efforts and rapid response mechanisms that minimize the window of vulnerability to cyber attacks. This integration empowers your cybersecurity teams to focus on strategic, high-level analyses while AI-driven systems handle the heavy lifting.
Enhancing Threat Detection
Artificial intelligence (AI) and machine learning (ML) are transforming the digital landscape of cybersecurity, particularly in enhancing threat detection. You’re facing an unprecedented challenge in safeguarding your digital assets due to the rapid evolution of cyber threats. Traditional methods of threat detection often fall short because they rely on known attack patterns. AI and ML change this dynamic by enabling systems to learn from vast amounts of data even if these patterns are not yet well-documented.
Automating Incident Response
Incident response, the next frontier in AI and cybersecurity integration, transforms the way you handle breaches. By automating incident response, AI systems can analyze event data and take necessary actions to mitigate risks. This proactive approach minimizes the time window for attackers to exploit vulnerabilities, reducing the impact of security breaches.
AI-powered incident response platforms can automate investigation workflows, rapidly and suggest appropriate remedial actions. These capabilities empower security teams to respond quickly, minimizing the impact of security breaches. For instance, AI can automatically block malicious IP addresses, shut down compromised systems to identify phishing attempts.
Conclusion
As you navigate the ever-evolving landscape of cybersecurity threats, integrating AI and ML becomes not just beneficial but essential. These technologies greatly enhance threat detection, automate processes, and enable rapid incident response. By leveraging AI’s ability to analyze vast amounts of data and predict vulnerabilities, you can proactively mitigate risks. However, it’s vital to address challenges such as data quality and adversarial attacks to guarantee AI-driven solutions remain effective and secure. Immediate adoption is imperative to protect against emerging threats.